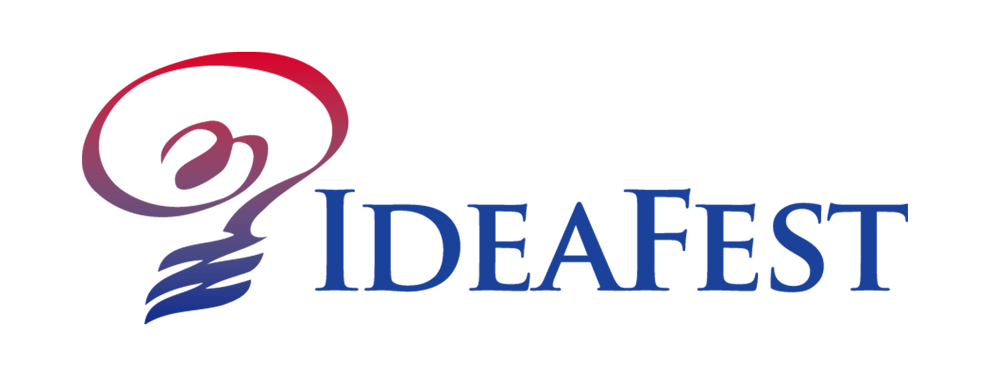
Title
Identifiability of Linear Compartmental Models: The Effect of Moving Inputs, Outputs, and Leaks
Document Type
Oral/Panel
Publication Date
5-2020
Disciplines
Mathematics
Abstract
A mathematical model is identifiable if its parameters can be recovered from data. Here we investigate, for linear compartmental models, whether local generic identifiability is preserved when parts of the model -- specifically, inputs, outputs, leaks, and edges -- are moved, added, or deleted. Our results are as follows. First, for certain catenary, cycle, and mammillary models, moving or deleting the leak preserves identifiability. Next, for cycle models with up to one leak, moving inputs or outputs preserves identifiability. Thus, every cycle model with up to one leak (and at least one input and at least one output) is identifiable. Finally, for certain cycle models with no leaks, adding specific edges again preserves identifiability. Our proofs, which are algebraic and combinatorial in nature, rely on results on elementary symmetric polynomials and the theory of input-output equations for linear compartmental models.
Research Area
Mathematic
Recommended Citation
Gerberding, Seth, "Identifiability of Linear Compartmental Models: The Effect of Moving Inputs, Outputs, and Leaks" (2020). IdeaFest. 100.
https://red.library.usd.edu/idea/100