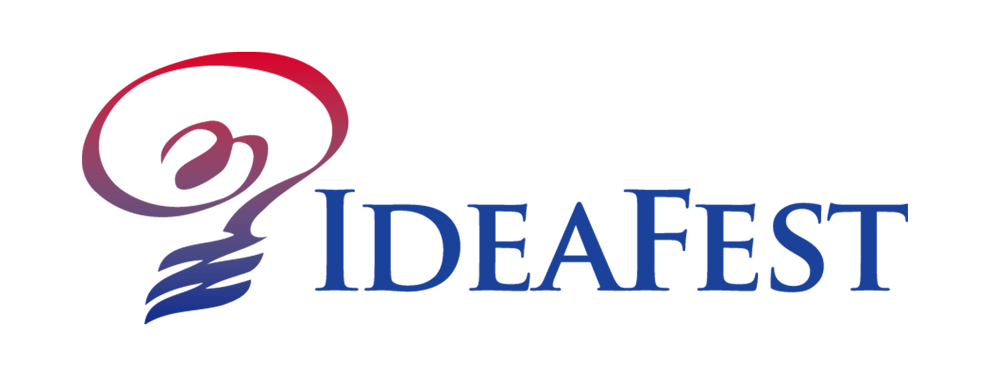
Document Type
Oral/Panel
Loading...
Publication Date
4-2021
Abstract
Background: As rapid growth of respiratory diseases is witnessed around the world; medical research field has gained interest in integrating audio signal analysis-based technique which aids timely diagnosis of respiratory ailments effortlessly in early stages of a respiratory dysfunction. Method: Our tool [1] distinguishes adventitious respiratory sounds using Linear Predictive Cepstral Coefficient (LPCC)-based feature [2] along with a Multi-Layer Perceptron (MLP)-based classifier. Results: When we applied our model on the largest publicly available ICBHI17 dataset [2] of size 6800+ clips we obtained the highest possible accuracy of 99.22% with an AUC value of 0.9993. Conclusion: Our results (accuracy 99.22%) outperformed established works in literature and other machine learning techniques and in future we will extend our research to identify nature and severity of infection just from the breath sounds.
First Advisor
KC Santosh
Research Area
Computer Science
Recommended Citation
Sreerama, Priyanka, "Automatic lung health screening using respiratory sounds" (2021). IdeaFest. 419.
https://red.library.usd.edu/idea/419